The Protein Folding Problem
Proteins are the architects of life, crafting every cellular process—from the rhythm of a heartbeat to the storage of memory. Yet, these molecular machines do not simply jump into action; they fold into intricate, three-dimensional structures that dictate their functions.7 For decades, predicting this process, known as the “protein folding problem,” was considered one of biology’s greatest unsolved mysteries.4,7
The protein folding problem lies in predicting how linear sequences of amino acids, the building blocks of proteins, fold into specific three-dimensional structures. The final structure is not random; it is determined by the sequence of amino acids and is crucial for protein structure. A protein’s shape dictates its ability to interact with other molecules and perform its role inside the body. These roles are incredibly diverse: Proteins can catalyze reactions, provide structural support, transport molecules, or regulate biochemical processes.2
Historically, solving this problem required labor-intensive techniques, such as X-ray crystallography and nuclear magnetic resonance spectroscopy, which, while effective, are time-consuming and are often limited to smaller proteins2. The complexity and variability of protein folding made this problem one of biology’s most challenging problems until recent advancements in computational biology transformed the field.2
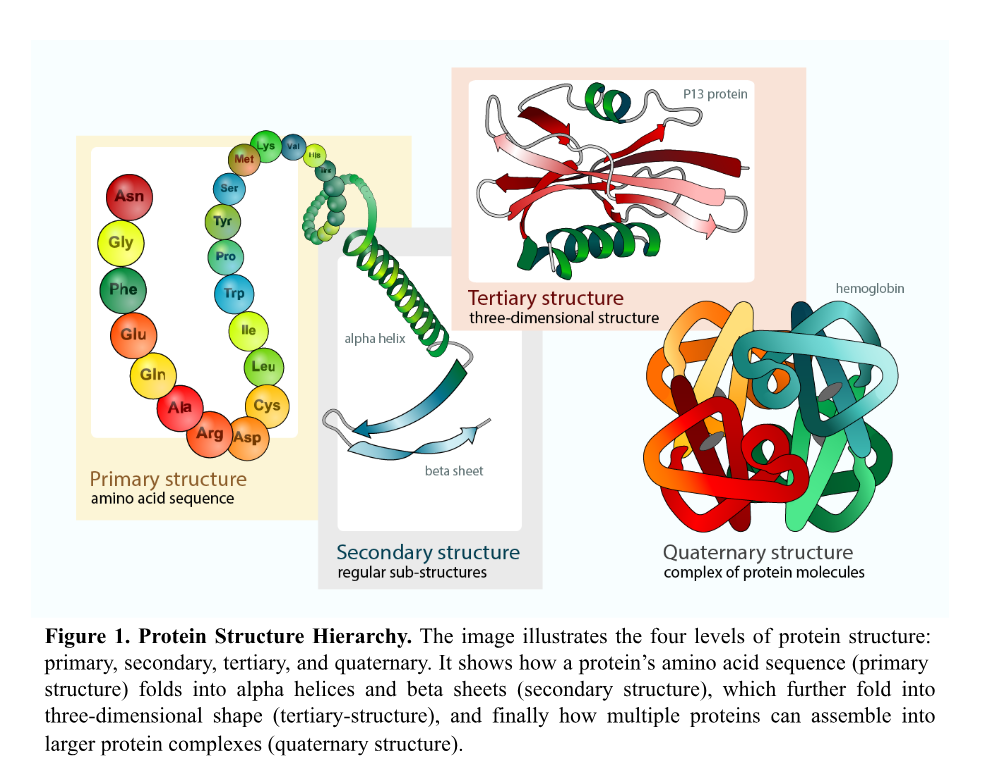
Enter AlphaFold (A-Fold), an artificial intelligence system developed by Google DeepMind that has revolutionized the field of protein prediction. The release of AlphaFold 1 in 2018 achieved the first significant breakthrough, but it was AlphaFold 2 in 2020 that revolutionized the field, predicting protein structures with a level of accuracy that was previously impossible.2,5
How AlphaFold Works
At its core, AlphaFold uses deep learning, a branch of artificial intelligence that mimics the brain’s neural networks, to predict a protein’s structure from its amino acid sequence. The process begins by searching genetic databases for similar protein sequences. Once it identifies these, A-Fold applies a technique called Multiple Sequence Alignment, a method that compares similar protein sequences to identify patterns and interactions between amino acids, which are crucial for protein folding2. By using MSA alignment, this reveals key relationships between different amino acids, known as residue-residue interactions, which are crucial for determining how the protein folds. Using this data, A-Fold predicts the final folded structure and generates a 3D model, where high-confidence regions are shown in blue and lower-confidence areas are highlighted in green. These confidence scores help researchers assess the accuracy of the prediction, providing valuable insights into the protein’s structure.2,5
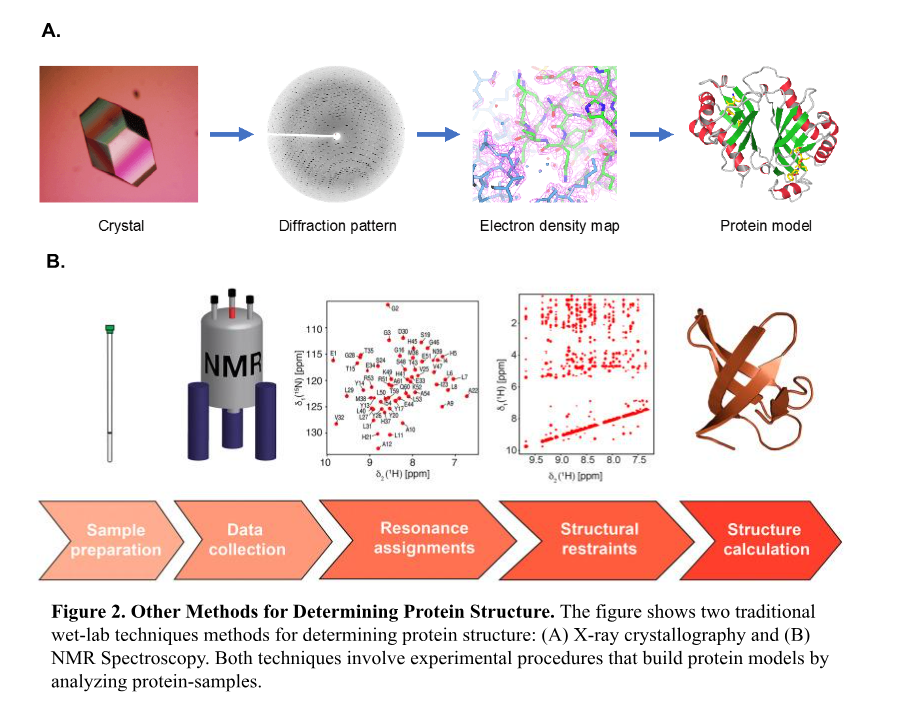
AlphaFold’s Role in Understanding Cardiovascular Disease
In cardiovascular disease, two proteins: Apolipoprotein B (ApoB) and Apolipoprotein E (ApoE), play critical roles in lipid metabolism, governing how fats like cholesterol are processed in the body. Misfolding of these proteins can lead to atherosclerosis, where fatty acids accumulate in arteries, raising heart attack and stroke risks.1
One of AlphaFold’s major contributions is its precise modeling of ApoB structure. A 2021 study led by Jumper et al. revealed critical details about ApoB’s specific binding sites and interactions with LDL cholesterol, showing how minor structural variances contribute to plaque buildup in arteries. Before A-Fold, scientists could only hypothesize on these molecular interactions, but now, with A-Fold’s high-resolution models, researchers can pinpoint key players that influence ApoB’s role in cardiovascular disease. This enables the design of drugs that target these precise areas, potentially blocking cholesterol’s harmful effects and reducing cardiovascular risk.1
Similarly, A-Fold’s accurate model of ApoE4 variant has shed light on its dual impact on cardiovascular disease and neurodegenerative disorders, like Alzheimer’s. By pinpointing structural features critical to ApoE4’s misfolding, A-Fold enables researchers to develop targeted therapies that can prevent the harmful effects associated with its misfolded form.1
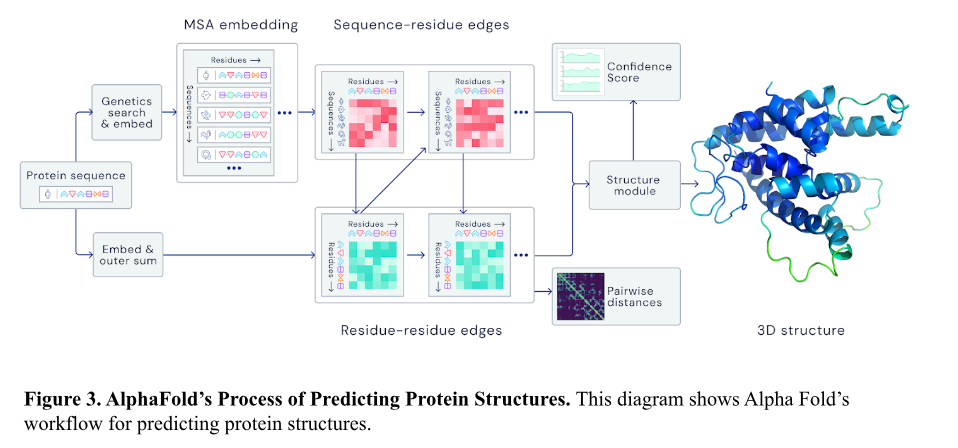
Limitations and Challenges of AlphaFold
While AlphaFold has revolutionized structural biology, it still faces some limitations. One key challenge is predicting how proteins interact with larger molecular complexes, such as DNA or RNA5 Although AlphaFold 3 has made significant progress in modeling protein interactions, it is not yet perfect in predicting these complex dynamics. Proteins rarely act alone, and understanding their interactions with other molecules is crucial for grasping their function.2
Protein flexibility is another area where A-Fold struggles. Proteins are not static; they constantly change shape depending on their environment and interactions with other molecules.2,5 While A-fold excels in predicting a protein’s most stable form, it has difficulty modeling proteins that can shift between multiple shapes. This behavior is essential in practical biological systems and represents an area for future improvement2
Finally, like any AI system, A-fold is only as good at the information it has been trained on. The model pulls from a huge database of known protein structures; however, there remain numerous proteins, particularly those found in rare biological conditions, that have yet to be fully explored. This gap may limit the accuracy of AF predictions, suggesting the need for more protein databases to improve its accuracy.5
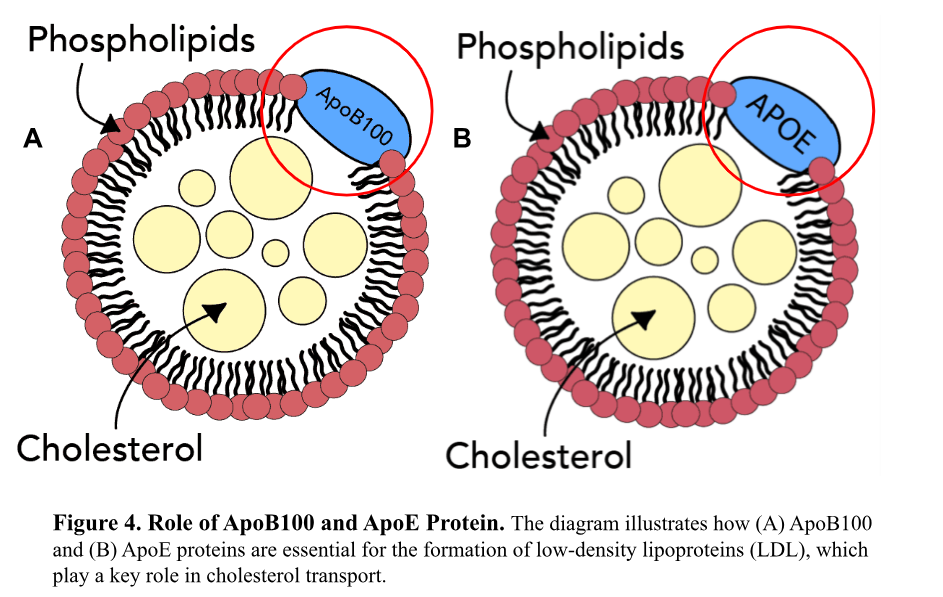
The Future of AlphaFold
Despite these limitations, AlphaFold’s development is far from finished. While it has revolutionized the prediction of static protein structures, the next challenge lies in understanding protein dynamics.2 Future iterations of A-fold may incorporate simulations that predict these movements, giving researchers a more nuanced and real-time understanding of protein behavior.2
As computational resources become more powerful and accessible, A-fold’s capabilities will most likely be made widely available through cloud platforms, expanding the access to this technology. This would allow researchers and scientists from across the globe, including those in resource-limited settings, to harness the power of A-fold for their work.2,6 With this increased accessibility, there could be a surge in breakthroughs in fields ranging from synthetic biology to rare diseases, where advanced protein modeling is limited.2,6
Acknowledgments
I would like to thank Hannah Rishik and Sophia Swartz, first and third year Molecular & Cellular Biology PhD students at the University of California, Berkeley, for generously reviewing my article and providing me with detailed and thoughtful feedback.
References
- Mahley, R.W. Apolipoprotein E: from cardiovascular disease to neurodegenerative disorders. J Mol Med 94, 739–746 (2016). https://doi.org/10.1007/s00109-016-1427-y
- Jumper, J., Evans, R., Pritzel, A. et al. Highly accurate protein structure prediction with AlphaFold. Nature 596, 583–589 (2021). https://doi.org/10.1038/s41586-021-03819-2
- Frankel, H. (2024, June 26). How AI revolutionized protein science but didn’t end it. Quanta Magazine. Retrieved from https://www.quantamagazine.org/how-ai-revolutionized-protein-science-but-didnt-end-it-20240626/
- Mirabello, C., Wallner, B., Nystedt, B. et al. Unmasking AlphaFold to integrate experiments and predictions in multimeric complexes. Nat Commun 15, 8724 (2024). https://doi.org/10.1038/s41467-024-52951-w
- Callaway, E. (2024). AlphaFold 3: How AI technology predicts protein dynamics. Nature. https://doi.org/10.1038/d41586-024-03423-0
- Staff, S. (2024). Powerful new AI software maps virtually any protein interaction in minutes. Science. Retrieved from https://www.science.org/content/article/powerful-new-ai-software-maps-virtually-any-protein-interaction-minutes
- Google. (2024). Google DeepMind introduces AlphaFold 3: Advancing AI for protein structure prediction. Google AI Blog. Retrieved from https://blog.google/technology/ai/google-deepmind-isomorphic-alphafold-3-ai-model/#life-molecules
Image References
- Header Image: DeepMind. (n.d.). A glimpse of the next generation of AlphaFold. DeepMind Blog. Retrieved from https://deepmind.google/discover/blog/a-glimpse-of-the-next-generation-of-alphafold/
- Figure 1: ResearchGate. (n.d.). Four levels of protein structure. ResearchGate. Retrieved from https://www.researchgate.net/figure/Four-levels-of-protein-structure_fig3_318422578
- Figure 2: Creative Biostructure. (n.d.). X-ray crystallography process: From crystal to protein model. Creative Biostructure. Retrieved from https://www.creative-biostructure.com/x-ray-crystallography-platform_60.htm
- Figure 3: O’Hare, R. (2021). AlphaFold 2: What’s behind the structure prediction miracle. Blopig Blog. Retrieved from https://www.blopig.com/blog/2021/07/alphafold-2-is-here-whats-behind-the-structure-prediction-miracle/
- Figure 4: Nebula Genomics. (n.d.). ApoE structure in lipoprotein. Nebula Genomics Blog. Retrieved from https://nebula.org/blog/apolipoprotein-e-apoe/